CS 712 Midterm paper 2024
Cs712 All Midterm Paper 2024 Till Now
Total papers shared = 26
8-6-24. 5.30pm
1-Apply natural join on a given table.
2-difference between PHF & DHF.
3. Discuss DHF with an example.
4. Scenario was given to identify types of access.
5. Define p2p, semi autonomous and heterogeneous with symbolic representations.
All questions were from “NAEEM WALI FILE”
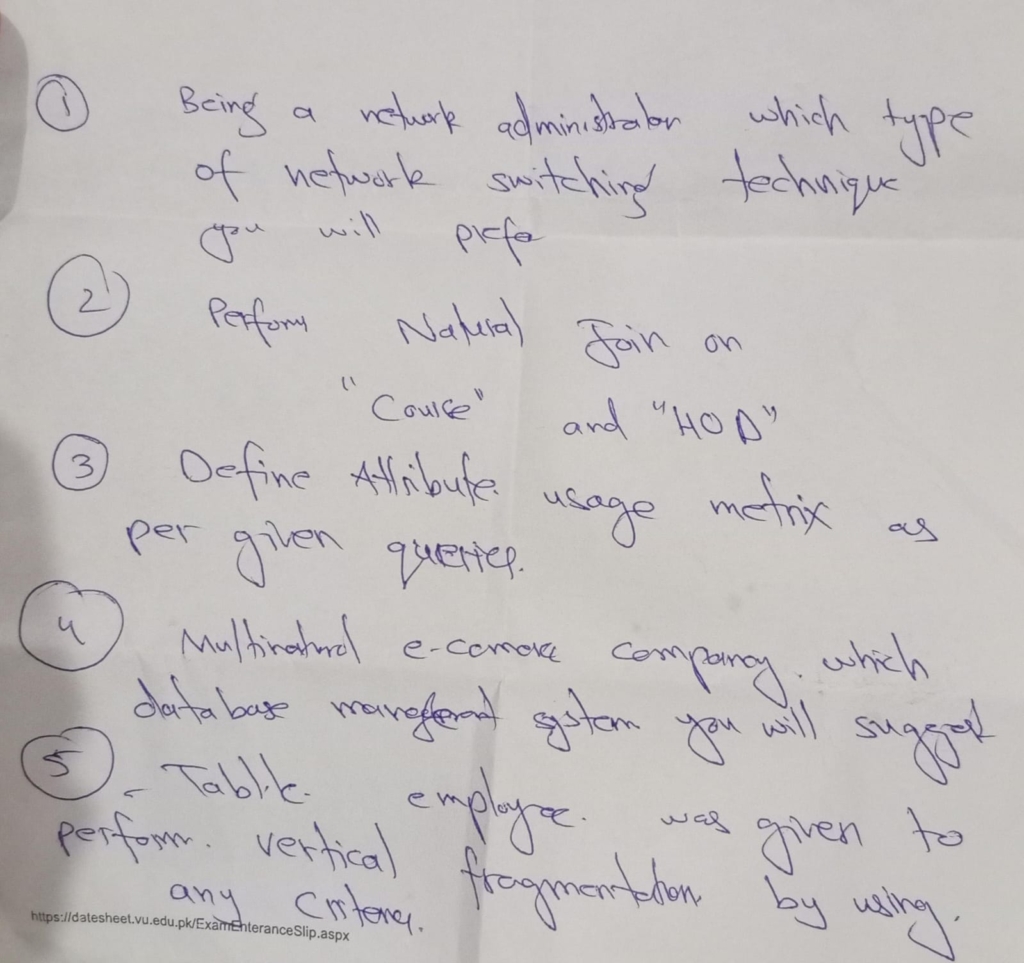
Today 2024 5 30
1.1st normal form
2. Textile mill
3. Diff PHF DHF4. Usage matrix
5. Vertical fragmentation
CS712 07.06.24
Top Down bottom up approach
Which topology should be in WAN
Completeness Define
Role of DBA, System Administrator, Enterprise Administrator
Characteristics of DBMS.
Today 5:30pm
Q1.(AoDoh1)
Q2.wan which topology
Q3.why DDbms
Q4.dhf with example
Q5 dhf long
CS712
01/06/24 – 11:00 AM
Q1. Difference between Primary and Derived Horizontal Fragmentation
Q2. Convert given Relation to 1NF (it had non atomic values in one of the columns)
Q3. Scenario was given for a Mill that had Centralised DB and wanted to expand over various countries and there was a need to convert to Dist. DB, question was in what way it could be done.
Q4. Relation was given, had to list 5 Simple Predicates
Q5. Affinity Attribute Matrix
Cs 712 1-06-2024 5:30pm
1. HOW you will convert Textile mill centralized DB to DDBMS
2. Difference b/w record based n semntic models
3. Degrees of fragmentation
4. Table was given n quries were given, it was to tell either quries are represent complete predicate or not
5. Scenario based question, as a DBA how will u convert ddba to multiple ddba without global schema
CS 712 Mid term 8:00
02-06-2024
1. Characteristics of DDBMS
2. Perform 2nd normal form on the table
3. Primary vs derived fragmentation
4. Verify Disjointness of horizontal fragmentation
5. Without global schema (10Marks)
2 June 24 10 AM Paper
1. 1.if hetrogenity in multi database exit what are approaches are used for give access to user
2. Scanio given in which and organization have multiple uni which dbms used
3. 3 completeness of hori zontal fragmentation
4. 3rd normal me table ko le kr Jana tha
5. Autonomy, Distribution and Heterogeneity
02-06-2024 Time= 12:30PM
1. table was given identify possible set of super key and primary key
2. How DDBMS handle following issues (a) Telecom cost & failure (b) Data availability (c) Reliability
3. Define degree of fragmentation
4. Table and 2 predicate were given . tell whether first predicate is complete with respect to second or not..
5. define semi autonomous, peer to peer and heterogeneous.. Also represent these architecture components symbolically
Cs712 mid term paper 2-6-2024 12.30 PM
1. Define rola DB administrator, Application administrator, Enterprise administrator
2. Which topology would u prefer in Wide Area Network. Write it’s characteristics and mode of operation
3. Scenario was given that U have 50,000 in account. U withdraw 20,000 but bank still shows 50,000. Its example of DDBMS, identify issue
4. Write steps of primary horizontal partitioning
5. Queries and access frequencies were given. Asked to compute attribute affinity matrix
1.as dba administrator which network switching technique you will use and why.
2.table was given to convert it in 2nd NF
3.Eshop company exist in different regions they using DDBS,why they are using DDBS,give 3 reasons
4.aik DHF k about question tha
5.give atleast 2 strategies for designing DDBS and give atleast one scenario where these strategies are applicable.
712 mid 5 30
Convert into first normal form. 5 marks
Advantage and disadvantages of fragmentation 5marks
Inputs for derived horizontal fragmentation and what are ownerand members 5 marks
Ecommerce db question which db will be used provide two solid reasons 5 marks
Peer to peer.semi autonoums and heterogenous db definition along with symbolic representation 10marks
712 4:00PM 2-6-2024
1. Define Derived Horizontal fragmentation with example
2. Someone want to access account from with in city , out of city and out of Country. What type of access it is?
3. give 3 reasons to use fragmentation
5. Fragment tables were given and have to tell which correctness horizontal fragmentation rules are violted in fragments. And how?
1 diff between DBMS n multi DBMS?
2 derived HF with example?
3 textile business wala
4 yad ni
5 table was given vertical fragmentation kr ni thi.
#CS712 3-6-2024
1. Bond energy algorithm steps
2. Third normal form. ek table given tha usko 3rd normal form mai convert karna tha
3. Scenario given tha ye batana tha k konsa Network best hai es k liye.
4. Scenario given tha aur ye batana tha k es k lye kona DBMS use karna chahye.
5. Attribute affinity matrix find karna tha.
712 paper 3-6-2024 at 5:30
1) explain (A0D1H0) and (A2D0H1) 5 marks
2) 2 tablets are given
Perform equi-join and show resultant table.
3) A scenario of a company was given or pocha gya tha k kon c dbms use krni chaye 2 reason btaien.
4) how completness of primary horizontal fragmentation kuch is tara ka sawal tham
5) distributed dbms ko designe krnay k liye 2 main approaches kon c hen or koi scenario btaien jahan ye apply ho sakay.
Cs 712
1.Degree of fragmentation
2.Table was given Define attributes affinity matrix
3.Scenario was given tell which dbms will be used
4.Two strategies for designing distributed database design
5.table was given tell which will be super key and primary key.
Today 11.00Am
1 – textile mill question
2 – advantages and disadvantages of fragmentation
3 – disjointness of horizontal fragmentation
4 – equijoin ka question tha tables given thy 2
5 – If you are DBA administrator, then how will you design multi-dbms without using global schema? (10)
1. PHF VS DHF
2. INPUTS FOR DHF
3. 3 REASON TO SHIFT FROM CENTRALIZED TO DDBMS
4. 3NF
5. MENTION MINTERMS FROM GIVEN TABLE
1. Define Semi-autonomous, peer to peer and heterogeneous. Also represent these architecture components symbolically.(10)
2. Explain (A0D1H0) and (A2D0H1)
3. Which topology would u prefer in Wide Area Network. Write it’s characteristics and mode of operation.
4. Table was given. Name the five predicates of it.
5. What are problems in establishing DDBMS
1.Mill question
2 . Scenerio Whether vertical fragmentation is correct
3. Multi DBMS design scenrio
4. A scenerio in which distributed design approaches
5. Yad nh
Mushkil tha mera to sary design
Global affinity matrix ka formula likha tha
Table given tha anomalies find karni thi
Or long mh affinity wala Q tha
1. Without global schema
2. Issues with DDBMS
3. Tell if query 1 is complete predicate with respect to query 2, with reasin
4. Which topology for a WAN network you will prefer. Write its features and transmission mode
5. Administrator roles
1-Table given tha convert 1st normal form
2-Inputs of DHF AND difference between owner and member
3-Which is responsible dB amninitrator
Enterprise database
4-Eshop or e-commerce convert to ddbms which reasons for this .
5-Calculate global affinity matrix ..query given and sites data given tha
1)A2D0H1,A0D1H1 architecture btana tha
2)EQUI-JOIN apply karna tha …table given tha
3)bank ki example thi..3 statement thi to access type btani thi,local ,remote ya global
4)vertical fragmentation di hue thi …justify karna tha k vertical fragmentation thek h ya ni
5) dba multi-database without global schema design kary…
Cs712 midterm 07.06.2024
Fragmentation advantages and disadvantages
Draw affinity Matrix 10 marks
Difference between record based and semantic models
Eshop secenario, why they choose DDBS
Draw attribute usage matrix
Cs 712 10-06-2024 5:30pm solved paper
- HOW you will convert Textile mill centralized DB to DDBMS
Ans: Converting a centralized database to a distributed database is a significant undertaking that requires careful planning and execution to ensure data consistency, availability, and scalability. Here’s a general approach to do this:
- Assessment and Planning:
- Understand the existing database schema, data model, and dependencies.
- Identify the reasons for moving to a distributed database. Common reasons include scalability, availability, and fault tolerance.
- Evaluate different distributed database options such as NoSQL databases like Cassandra, MongoDB, or distributed SQL databases like Google Spanner, CockroachDB, etc.
- Determine the requirements and constraints of the new distributed database, such as data consistency, replication, partitioning, and sharding strategies.
- Data Modeling:
- Redesign the database schema if necessary to fit the requirements of the distributed database.
- Consider data partitioning and sharding strategies to distribute data across multiple nodes.
- Ensure that the new data model supports efficient querying and transactions in a distributed environment.
- Data Migration:
- Develop a migration plan to transfer data from the centralized database to the distributed database.
- Use ETL (Extract, Transform, Load) tools or custom scripts to migrate data while ensuring data integrity and consistency.
- Test the migration process thoroughly to identify and address any issues or inconsistencies.
- Application Refactoring:
- Modify the application code to work with the new distributed database APIs and query languages.
- Update database access patterns to take advantage of distributed features such as sharding and replication.
- Implement error handling and retry mechanisms to handle distributed system failures and network partitions.
- Testing:
- Perform comprehensive testing of the distributed database setup under various conditions, including high load, network failures, and node failures.
- Test data consistency, availability, and performance to ensure that the new distributed database meets the requirements.
- Deployment:
- Deploy the distributed database in a production environment, following best practices for security, monitoring, and maintenance.
- Monitor the performance and health of the distributed database continuously, and make adjustments as necessary.
- Training and Documentation:
- Provide training to the operations team on managing and maintaining the distributed database.
- Document the architecture, configuration, and operational procedures for the distributed database.
- Optimization and Continuous Improvement:
- Continuously monitor the performance and scalability of the distributed database and make optimizations as needed.
- Stay updated with new features and best practices in distributed database technologies to leverage them for further improvements.
Throughout the process, it’s essential to involve stakeholders from different teams, including database administrators, developers, and operations, to ensure a smooth transition to the distributed database. Additionally, consider seeking assistance from experienced database consultants or vendors if needed.
- Difference b/w record based n semantic models
Ans: Data Model: a set of tools or constructs that are used to design a database. There are two major categories of data models.
- Record based/Legacy data models.
data models that have relatively less constructs and the identification of records (defining key) is the responsibility of the user. This type of data models are Network, Hierarchical and Relational Data Models.
- Semantic data models
data models are the ones that have more constructs, so they are semantically rich, moreover the identification of records is managed by the system. Examples of such data models are Entity-Relationship and Object-Oriented data models.
- Degrees of fragmentation
Ans: The degree of fragmentation in a distributed database refers to the extent to which data is divided or distributed across multiple nodes or partitions within the database system. Fragmentation can occur at different levels: data fragmentation, location fragmentation, and network fragmentation.
- Data Fragmentation:
- Data fragmentation refers to the division of data objects (such as tables or records) into smaller pieces or fragments that are distributed across multiple nodes or partitions.
- There are two primary types of data fragmentation:
- Horizontal fragmentation: Divides a table into multiple fragments, with each fragment containing a subset of rows.
- Vertical fragmentation: Divides a table into multiple fragments, with each fragment containing a subset of columns.
- Location Fragmentation:
- Location fragmentation refers to the distribution of data fragments across different physical locations or nodes within the distributed database system.
- Network Fragmentation:
- Network fragmentation refers to the distribution of data fragments across different network segments or connections within the distributed database system.
The degree of fragmentation in a distributed database can impact various aspects of the system, including performance, scalability, and fault tolerance. A higher degree of fragmentation can provide better load balancing, improved parallelism, and enhanced fault tolerance by distributing data and workload across multiple nodes. However, it can also introduce challenges such as increased network overhead, data consistency issues, and complexity in data management and querying.
4. Table was given n queries were given, it was to tell either queries are represent complete predicate or not
5. Scenario based question, as a DBA how will u convert ddba to multiple ddba without global schema
Ans: Converting a centralized database administrator (DBA) role to multiple distributed database administrators (DBAs) without a global schema involves decentralizing the management and administration of the database across multiple distributed nodes or partitions. Here’s how you might approach this:
- Identify Distributed Database Nodes:
- Determine the number of distributed nodes or partitions in the distributed database system.
- Each node may represent a separate physical server, data center, or logical partition within the distributed database.
- Assign DBA Responsibilities:
- Divide the DBA responsibilities among the distributed database nodes based on factors such as geographical location, workload distribution, or functional specialization.
- For example, you might assign specific nodes or partitions to handle database administration tasks such as backup and recovery, performance tuning, security management, or schema design.
- Establish Local Administration Teams:
- Form local administration teams consisting of DBAs responsible for managing and maintaining the databases within each distributed node or partition.
- Each local administration team should have the necessary skills and expertise to handle the DBA responsibilities assigned to them.
- Define Communication and Collaboration Channels:
- Establish communication and collaboration channels between the local administration teams to facilitate coordination and knowledge sharing.
- This might include regular meetings, online forums, documentation repositories, or collaboration tools for sharing best practices, troubleshooting issues, and coordinating database changes.
- Implement Role-Based Access Control (RBAC):
- Define role-based access control policies to restrict access to specific database resources and functionalities based on the responsibilities assigned to each local administration team.
- RBAC ensures that DBAs only have access to the database objects and operations relevant to their assigned roles, reducing the risk of unauthorized access or accidental changes.
- Provide Training and Support:
- Offer training and support to the local administration teams to ensure they have the necessary skills and knowledge to effectively perform their DBA responsibilities.
- This might include technical training on database management tools and technologies, as well as ongoing support and mentoring from senior DBAs or subject matter experts.
- Implement Monitoring and Alerting:
- Deploy monitoring and alerting systems to track the health, performance, and availability of the distributed database nodes and partitions.
- Monitoring tools should provide visibility into key metrics and performance indicators to detect issues proactively and alert the appropriate local administration teams for resolution.
- Review and Iterate:
- Regularly review the distributed database administration model to assess its effectiveness, identify areas for improvement, and address any challenges or bottlenecks.
- Iterate on the model based on feedback from the local administration teams and changes in the distributed database environment.
By decentralizing the DBA role across multiple distributed nodes or partitions and establishing local administration teams, you can effectively manage and maintain the distributed database system without relying on a global schema or centralized administration model.
Top of Form
Bottom of Form
CS712
01/06/24 – 11:00 AM
Q1. Difference between Primary and Derived Horizontal Fragmentation
Ans: Primary horizontal fragmentation and derived horizontal fragmentation are two approaches used in distributed databases to partition a table horizontally into smaller subsets or fragments. Here’s how they differ:
- Primary Horizontal Fragmentation:
- Definition: Primary horizontal fragmentation involves explicitly dividing a table into multiple fragments based on a fragmentation predicate.
- Fragmentation Predicate: Each fragment is defined by a fragmentation predicate, which specifies a condition or criterion for selecting rows to be included in the fragment.
- Example: For a table of customer records, primary horizontal fragmentation might involve dividing the table into fragments based on the geographical location of customers, where each fragment contains records for customers from a specific region or country.
Derived Horizontal Fragmentation:
- Definition: Derived horizontal fragmentation involves dynamically deriving fragments based on the data distribution or access patterns of the table.
- Derived from Data Distribution: Fragments are derived based on statistical analysis of the data distribution, such as clustering similar rows together to form fragments.
The main difference between primary horizontal fragmentation and derived horizontal fragmentation lies in how fragments are defined and managed. Primary fragmentation involves explicit definition of fragmentation predicates by administrators, while derived fragmentation dynamically derives fragments based on data distribution and access patterns. Both approaches aim to improve distributed database performance and scalability by partitioning tables into smaller, more manageable fragments.
Q2. Convert given Relation to 1NF (it had non atomic values in one of the columns)
Q3. Scenario was given for a Mill that had Centralised DB and wanted to expand over various countries and there was a need to convert to Dist. DB, question was in what way it could be done.
Q4. Relation was given, had to list 5 Simple Predicates
In the context of database management, predicates play a crucial role in defining conditions for selecting or filtering data from tables. Here’s a breakdown of what predicates are and how they’re used:
- Definition:
- A predicate is a logical condition or expression that evaluates to true, false, or unknown.
- Predicates are used in SQL queries to filter rows from tables based on specific criteria.
- Predicates can involve comparisons, logical operators, and functions to define complex conditions.
- Types of Predicates:
- Comparison Predicates: These involve comparing values using operators such as “=”, “<“, “>”, “<=”, “>=”, “!=”, etc.
- Logical Predicates: These involve logical operations such as AND, OR, NOT to combine multiple conditions.
- Membership Predicates: These involve checking if a value is within a specified set or range, using operators like IN, BETWEEN, LIKE, etc.
- NULL-Related Predicates: These involve checking for NULL values using operators like IS NULL and IS NOT NULL.
- Pattern Matching Predicates: These involve searching for patterns within strings using operators like LIKE and regular expressions.
- Use Cases:
- Data Retrieval: Predicates are commonly used in SELECT statements to retrieve specific rows from tables based on defined conditions.
- Data Modification: Predicates can also be used in UPDATE and DELETE statements to modify or remove rows that match certain criteria.
- Data Integrity: Predicates can enforce constraints and ensure data integrity by specifying conditions for INSERT and UPDATE operations.
- Join Conditions: Predicates are essential in specifying join conditions when combining data from multiple tables in a query.
- Examples:
- SELECT * FROM Employees WHERE Salary > 50000;
- This query retrieves all employee records where the salary is greater than 50,000.
- UPDATE Products SET Quantity = Quantity – 1 WHERE ProductID = 1001;
- This query decrements the quantity of a product with ID 1001 by 1.
- DELETE FROM Orders WHERE OrderDate < ‘2022-01-01’;
- This query deletes all orders placed before January 1, 2022.
- SELECT * FROM Employees WHERE Salary > 50000;
Predicates are fundamental to querying and manipulating data in databases, enabling precise control over which data is accessed, modified, or deleted based on specified conditions. Understanding how to use predicates effectively is essential for efficient database management and application development.
Q5. Affinity Attribute Matrix
Ans: An affinity attribute matrix, also known as an affinity matrix or simply a similarity matrix, is a data structure used in various fields such as machine learning, clustering, recommendation systems, and network analysis. It represents the similarity or affinity between pairs of entities in a dataset. Here’s how it works:
- Definition:
- An affinity attribute matrix is typically a square matrix where each row and column represent the same set of entities or items in a dataset.
- The elements of the matrix represent the similarity or affinity between pairs of entities. This similarity can be measured using various metrics, such as distance, correlation, or cosine similarity.
- The diagonal elements of the matrix usually represent the self-similarity of each entity, which is often set to a predefined value (e.g., 1 or 0) depending on the context.
- Construction:
- The affinity matrix is constructed based on the attributes or features of the entities in the dataset.
- For example, in a recommendation system, the attributes might represent user preferences or item characteristics, and the affinity between users or items is computed based on the similarity of these attributes.
- In network analysis, the affinity between nodes in a network can be computed based on topological properties such as connectivity or structural similarity.
- Applications:
- Clustering: Affinity matrices are commonly used in clustering algorithms such as spectral clustering or affinity propagation, where the similarity between data points is used to group them into clusters.
- Recommendation Systems: In collaborative filtering-based recommendation systems, affinity matrices are used to compute similarities between users or items to make personalized recommendations.
- Network Analysis: Affinity matrices are used in network analysis to quantify relationships between nodes in a network, such as in community detection or link prediction tasks.
- Dimensionality Reduction: Affinity matrices are sometimes used as input to dimensionality reduction techniques like multi-dimensional scaling (MDS) or t-distributed stochastic neighbor embedding (t-SNE) to visualize high-dimensional data in lower dimensions while preserving the underlying structure.
- Example:
- Suppose we have a dataset of users and items, where each user has rated multiple items on a scale of 1 to 5. We can construct an affinity matrix where each element represents the similarity between pairs of users based on their rating patterns. Higher similarity values indicate that users have similar preferences in terms of item ratings.
Overall, affinity attribute matrices are versatile data structures that enable the representation and analysis of pairwise relationships between entities in a dataset, facilitating various analytical tasks such as clustering, recommendation, and network analysis.